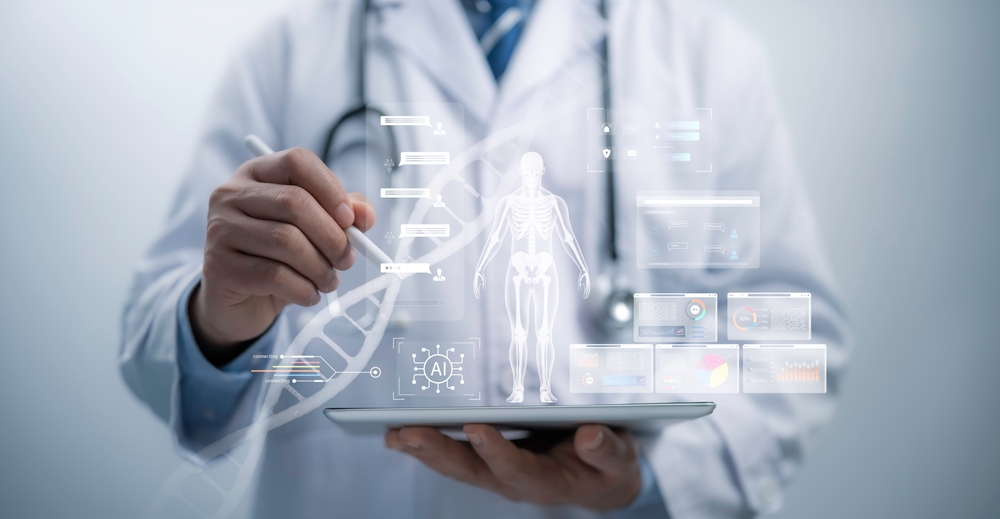
Artificial intelligence has become a buzzword—every article, news bulletin, podcast, and other digital media seems to mention it.
Looking back at the past decade, the field of AI has been growing rapidly, and perhaps its crescendo was the mainstream explosion of ChatGPT, a generative AI chatbot that was the fastest-growing consumer application software by January 2023. It is credited with starting the AI boom, a trend we still see today as investors pour millions of dollars into generative AI.
The life sciences industry, albeit having taken a more cautious approach, has not been left behind. According to a report from Grand View Research, the global life sciences AI market was valued at $1.3 billion in 2020 and is expected to grow at an annual rate of 11.1% from 2023 to 2030.
While the potential for AI to save lives in areas like treatment and drug discovery is undeniable, one thing is without a doubt—the risks of artificial intelligence are very much real.
Understanding The Impact of AI on Life Sciences
The future is AI; there’s no doubt about that. It’s already reshaping the life sciences industry on multifaceted fronts, with drug discovery, known for its cost, length, and uncertainty, leading the way.
Today, life science companies use AI-powered tools and platforms to identify and map disease pathways and investigate complex protein interactions, a strategy that is paving the way for developing new and effective drugs and treatments.
Chemistry42, a proprietary generative AI-powered platform by Insilico Medicine, exemplifies the potential of this technology in the life sciences industry. Running on 42 generative algorithms, Insilico used the AI-powered tool during every stage of the drug discovery process to determine molecules that a drug compound could target.
Such drug discovery processes traditionally require over $400 million and up to six years to expedite. However, Insilico accomplished this pharma achievement for $40 million in two years using Chemistry42, a remarkable feat showing AI’s power in life sciences.
This is just one of the remarkable examples of AI’s transformative power. In areas such as personalized medicine, AI is taking centre stage helping medical professionals develop more tailored treatment plans and accurate diagnostics.
AI in Life Sciences Challenges
Even with all being said, the life science market has generally taken a more cautious approach to AI. In fact, most AI-assisted achievements in this niche are still in their early stages, and for good reason. Here are AI technology’s most significant risks for the life sciences market.
Data Quality and Bias
An AI model is only as strong as the data it is trained on. After all, data is the lifeblood of any AI system; without it, AI algorithms cannot learn to make accurate predictions.
That being said, AI algorithms trained on incomplete datasets can make biased decisions. In contrast, those trained on inaccurate information can make equally incorrect conclusions, a risk that has manifested in real-world AI failures.
A notable example is Apple’s Credit AI algorithm which began after popular software developer David Heinemier complained on Twitter that the credit line offered by his Apple card was 20 times higher than his wife’s, even though they filed joint tax returns and he had the worse credit score.
After his tweet went viral, more Apple customers responded with similar complaints, accusing the Apple Credit AI algorithm of gender bias, prompting the New York Department of Financial Services to investigate Goldman Sachs, the company responsible for running Apple Card.
This is a risk the life sciences industry should take very seriously as machine learning models and algorithms used in clinical trials and drug discovery heavily rely on medical data like electronic health records. Suppose such tools are trained on biased and incomplete data. The effects could be dangerous, potentially compromising patient safety.
Cybersecurity Threats
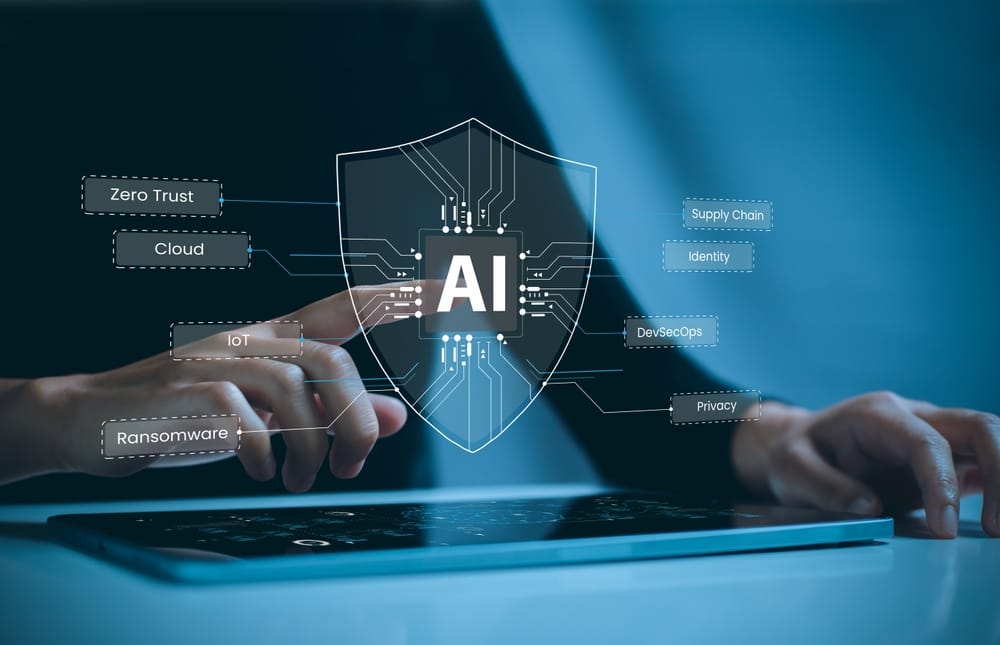
AI is a double-edged sword, a nature exemplified by its adoption in the hacker world. As this technology advances, the access barrier for criminals continues to decrease, allowing them to leverage AI-powered cyber-attack techniques.
Deepfakes are a prime example of how AI is reshaping cybercrime. Recently, a finance worker at a multinational firm in Hong Kong was duped into remitting $25 million after a video call with people they thought to be colleagues, only to realize they were deepfake recreations.
The risk of an AI-powered data breach or social engineering scam such above is very real for life sciences companies. In 2021 alone, more than 40 million patient records were compromised in US data breaches.
However, AI being a two-faced coin, presents an advantage. According to a report from the World Economic Forum, AI technology can be used to defend and prevent these attacks.
For example, artificial intelligence systems have a high malware detection accuracy. AI algorithms can be designed to analyze systems for questionable activity, pointing to the possibility of a data breach.
Technology and Performance Failures
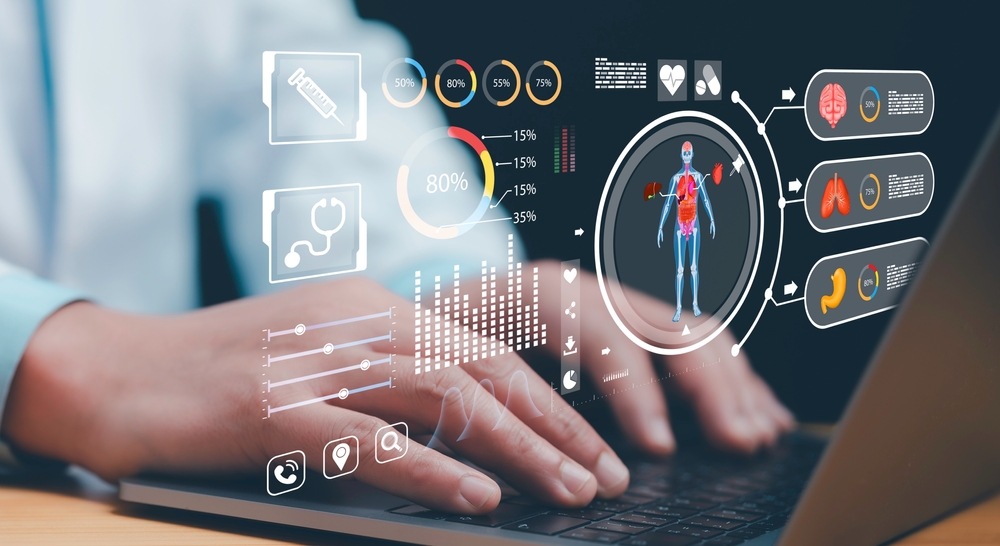
As life sciences companies continue to integrate AI applications in core operations, there’s always a risk of performance failures when AI models’ capabilities are exaggerated or when the output is inaccurate.
Take the case of Watson for Oncology, an IBM AI-powered tool designed to help doctors provide treatment recommendations for cancer patients based on literature from past cancer cases.
Internal documents from IBM revealed that the tool recommended unsafe and inaccurate treatments to doctors in what was to become an AI performance failure whose causes were as complex as they were nuanced.
As such, life science companies need to take the risk of AI performance failures very seriously. While natural language processing technology can extract data from clinical trials and other areas, it is crucial to ascertain the validity of AI recommendations and data, as these models are prone to making errors.
Conclusion
We have come to the end of our discussion of the dangers of artificial intelligence in the life sciences market. It’s clear that integrating this promising technology necessitates caution from all involved stakeholders, and as a tightly regulated industry, we expect to see policy changes soon.
While AI has proven to be a game-changer so far, we have yet to see its full capability because integration is still in its infancy. Looking at cases such as Insilico Medicine, a biomedical company that harnessed the power of artificial intelligence to create a new drug-like molecule 15 times faster than average, it is clear that the future is indeed AI.
However, as life science companies continue to utilize AI technology in drug discovery, clinical trials, and personalized medicine, the associated risks cannot be ignored. After all, with algorithms and machine learning models, the tool is only as good as the data it’s trained on.
As such, the risk of data bias leading to inaccurate conclusions putting patient lives at risk, is very much real. With examples such as Oncology by Watson to look back on, governments, private companies, and healthcare institutions must collaborate to ensure medical data systems are transparent and unbiased. Additionally, life science companies must reorganize their cybersecurity measures and keep workers up to date with current trends. As AI advances, distinguishing between synthetic and human-generated digital media is